Outcome
In Sweat I laid out the strategy behind the difficult segmentation of their persona base with a sound research methodology. Not only this allowed the company departments like support, engineering and marketing connect with the user base, but Sweat’s Personas played pivotal to enhance business performance and reduce churn opportunistically.
Method: Ideation, research and data
When I arrived to the company, Sweat described its user base as you would imagine; women in certain countries within a typical age range for a health and fitness app, which was a problem, since it was incredibly broad and unspecific to be actionable. This made it difficult for the company departments to connect meaningfully with its user base.
As compared to other apps, Sweat’s purpose is very specific, which is to workout, meaning that all personas would try to accomplish, in one way or another, the same goal, which made it difficult to segment.
My role involved envisioning a research method that would allow the company to design targeted user surveys to validate or discard hypotheses made about our user base, and to develop communication engagement plans and content strategy.
Divergence
I created and led a task-group involving the Customer Care, User Experience and Marketing managers together with the SME.
The first assignment involved individually coming up with educated guesses of who should we be designing for.
During the readout and introduction of the 16 personas we created, we found no meaningful ways to describe simply our top 3 or 4 personas.
Emergence
Led an affinity diagram exercise with the same group, with the goal to identify patterns in personas’ pain-points and goals, which allowed us to identify four key traits a-la BJ Fogg: Disposable Income ($) , Motivation (M), Time Availability (T) and Sociability (S).
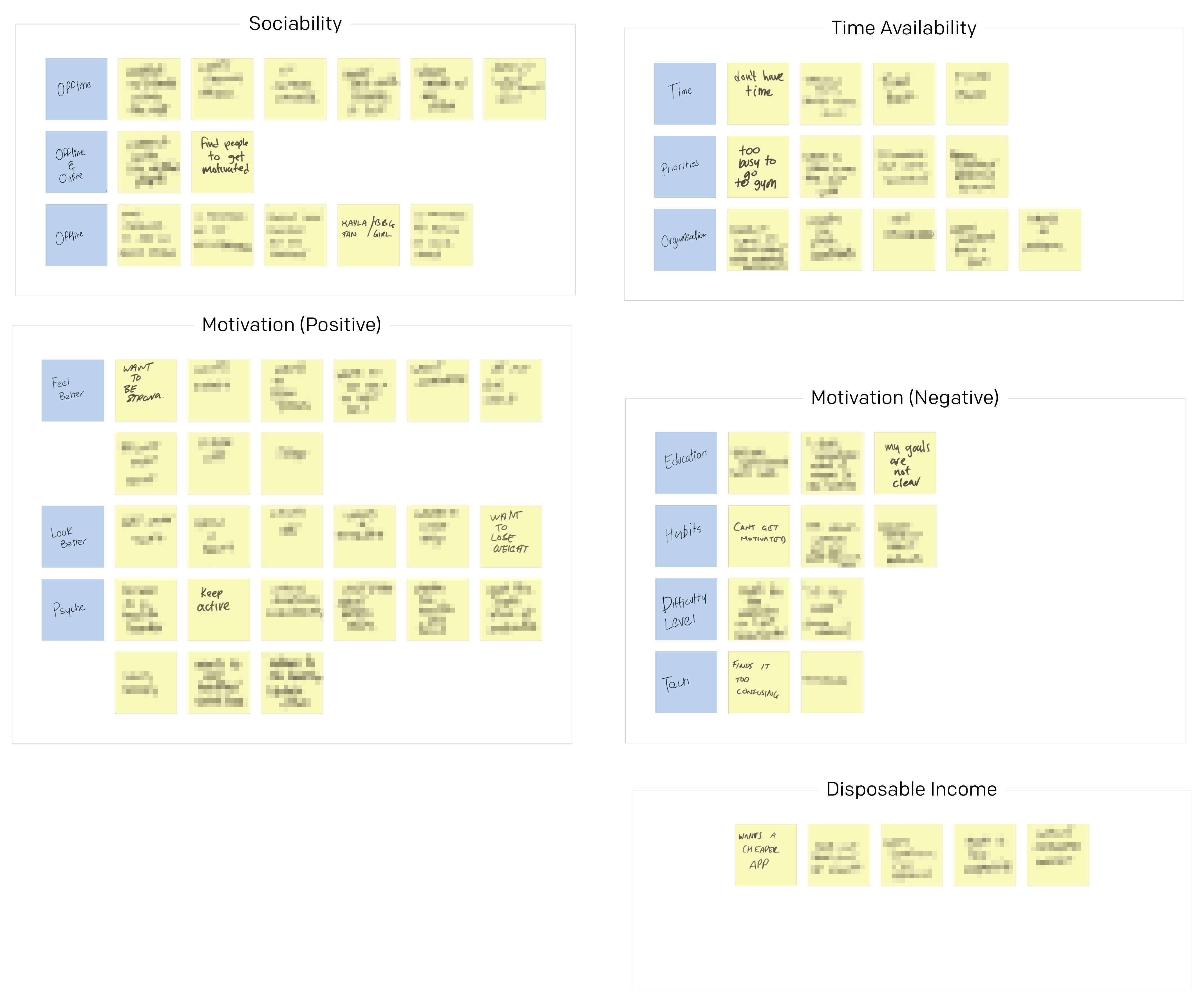
Convergence
After analyzing these conventions, I facilitated our SME group to come to an agreement to which of these trait combinations made sense based on the absence [N], presence [Y], or irrelevancy [?] of these key traits, as this gave us very specific leverage with certain kind of audience we wanted to connect with. For example in the table below (fictitious), persona Jennie, is a kind of user with a lot of time in her hands, regardless of how much money, motivation or sociable she is.
So we went from:

to:
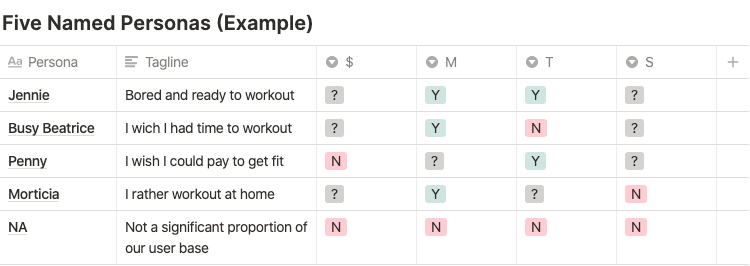

We identified, different several key states in which these groups could exist:
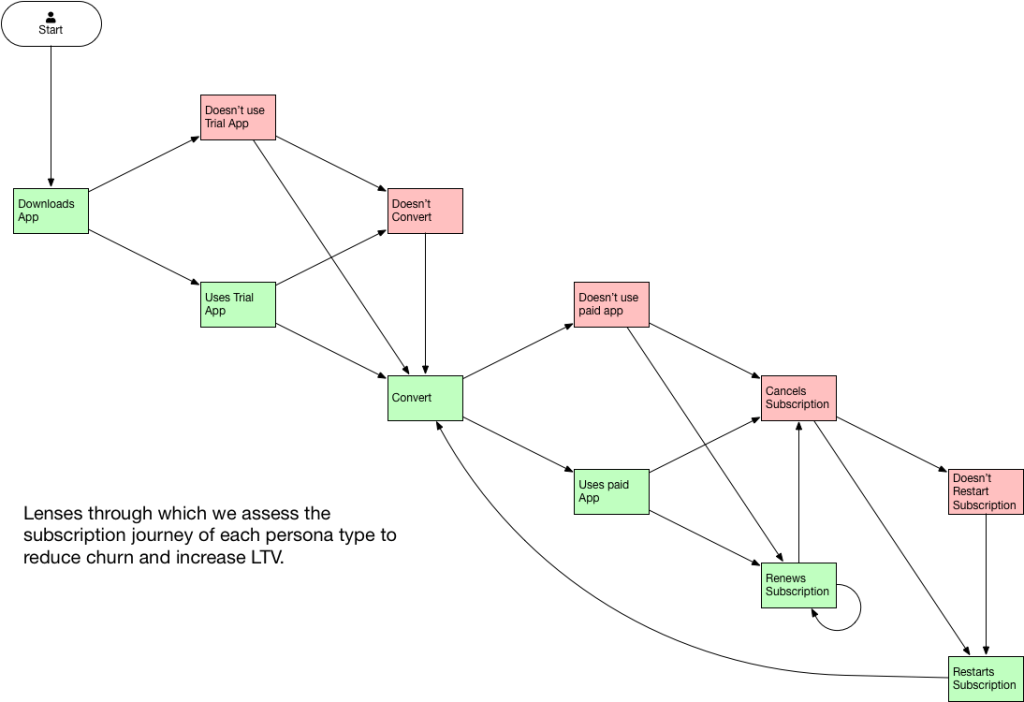
Data, data, data!
In Sweat this became the foundation for future research regarding personas, which included a series of in-app and post-app surveys that would allow us to automatically profile our users in the CRM.
This strategy included specific timed engagement plans to maximize results.
We determined the confidence level and confidence interval to get the send out the least amount of surveys and get statistically valid data.